[ML/Sklearn] ROC Curve visualization
Joonas' Note
[ML/Sklearn] ROC Curve visualization 본문
ROC(Receiver Operating Characteristic) curve는 TPR(True Positive Rate)와 FPR(False Positive Rate)의 변화값을 보는 데 이용한다. 분류가 잘 되었는 지 확인할 수 있는 성능 지표 중 하나이다.
sklearn에서는 이것을 차트로 그려주는 RocCurveDisplay 모듈이 있다.
3가지 방법이 있는데, 직접 TPR과 FPR을 넘겨서 그리는 방법은 문서에서 권장하지 않고, 모델로부터 그리는 from_estimator와, 정답 레이블과 예측 레이블을 넘겨서 그리는 from_predictions를 권장하고 있다.
import matplotlib.pyplot as plt
from sklearn.datasets import make_classification
from sklearn.metrics import RocCurveDisplay
from sklearn.model_selection import train_test_split
from sklearn.svm import SVC
X, y = make_classification(random_state=0)
X_train, X_test, y_train, y_test = train_test_split(X, y)
clf = SVC(random_state=0).fit(X_train, y_train)
y_pred = clf.decision_function(X_test)
fig, axes = plt.subplots(1, 2, figsize=(20,5))
axes[0].set_title('ROC-AUC from estimator')
axes[1].set_title('ROC-AUC from predictions')
RocCurveDisplay.from_estimator(clf, X_test, y_test, ax=axes[0])
RocCurveDisplay.from_predictions(y_test, y_pred, ax=axes[1])
plt.show()
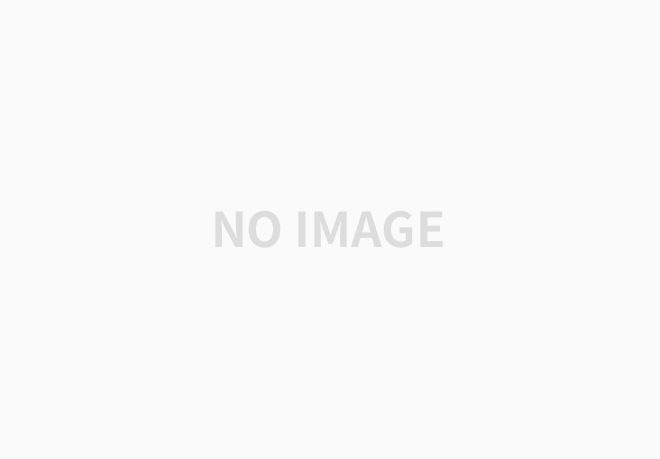
predictions로 넘기는 y_pred는 2가지 방법이 있다. 위처럼 decision_function()의 결과값을 넘기는 방법이 첫 번째이고.
두 번째는 y_pred_proba 함수의 결과값을 사용하는 것이다. 이 함수는 모델에 따라 별도의 설정이 필요할 수도 있고, 존재하지 않을 수 있다. (LinearSVC 등)
# Method 1
y_pred = clf.decision_function(X_test)
RocCurveDisplay.from_predictions(y_test, y_pred)
# Method 2
y_pred_proba = clf.predict_proba(X_test)
RocCurveDisplay.from_predictions(y_test, y_pred_proba[:,1])
y_pred_proba[:,1] 로 한쪽 열만 슬라이싱하는 이유는, 어차피 합쳐서 1.0 이고, 차트에는 둘이 구분될 수 있도록 점을 표시하는 것이기 때문이다.
'AI > 머신러닝' 카테고리의 다른 글
한국 가요(1964~2023)를 주제별로 모아보기 (0) | 2024.03.03 |
---|---|
[ML] 사이킷런(scikit-learn) 클러스터링 비교 (0) | 2022.05.29 |
Content-based File Format Detection (파일 확장자 예측) (0) | 2022.05.18 |